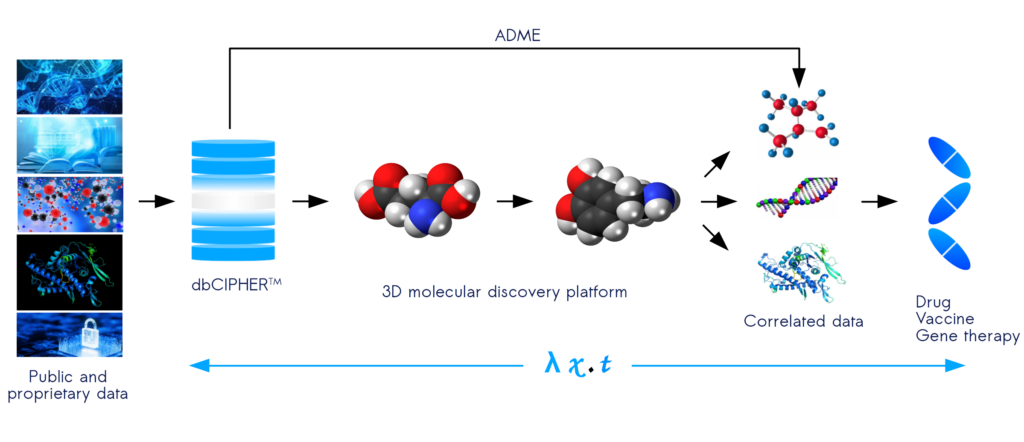
We are developing a CPU and GPU accelerated computing platform called, dbCIPHERTM (Correlating Information using Patterns of Hidden Ergodic Rules), that will revolutionize the way molecular, genomic, proteomic, and metabolomic data is represented, searched, and deciphered for creating a targeted drug discovery pipeline. This next-generation technology will allow organizations to pave the way to creating new molecules based on fundamental atomic and molecular theories in chemistry. Our proprietary computing architecture creates a treasure chest of molecular models that work together to synthesize a new language for molecular generation that will supercharge the next wave in drug discovery and precision medicine. Our system creates mathematical sentences from computed data structures that are then filtered using pharmacokenetics, pharmacodynamics, and ADME to target the newly designed molecules for selected optimization. Stay tuned for a detailed explanation of how dbCIPHERTM will unlock a superhighway of intelligent hidden information for turbocharging a new era in drug discovery.
Our in-silico pipeline combines supervised learning and reinforcement learning for generation molecules. Our reinforcement learning steps for molecular generation involves the use of a dynamical space in the form of the environment which represents the system of possible molecules where the molecular design agent interacts to create molecules. The actions are chemical transformations such as bond formation. The states represent the current partial molecular structure. Rather than deal with a SMILES or molecular graph, we use the three dimensional structure and perform energy calculation to find the most stable configurations using a Monte Carlo method coupled to a dynamical system and perform molecular energy minimization simulations that involves over time to find the most stable 3D structure of a molecule by iteratively adjusting atomic positions and minimizing the potential energy. Cipherica’s molecular generation steps evolve under an ergodic process that describes a function for representing what future states follow from the current state; the core of our proprietary reinforcement learning algorithm.