At Cipherica, we are focused on molecular generation at the in-silico stage of a biotech drug discovery pipeline. In biotech, your pipeline heavily influences the success of the drug development process. Our discovery platform, dbCIPHERTM, can generate lead compounds that potentially have a greater chance of becoming a successful drug. Historically, this process has taken many years along with large sums of investment money to take a potential drug across the finish line and into the clinic. Biotech is experiencing a resurgence and is extremely ripe for funding. However, biotech is an interesting industry. It’s the only market with devastating failures that largely outnumber the wins. Yet, it’s the only industry with the primary goal of improving and saving lives. This unique combination of risk and fascination draws investors to biotech. The global artificial intelligence in drug discovery market size was estimated at USD 1.9 billion in 2024, and is expected to expand at a CAGR of 29.7% from 2025 to 2030. The demand for AI-powered solutions in drug discovery is rising due to the need for novel drug therapies, increased manufacturing capacities in the life sciences industry, and technological advancements. Between 2019 and up to the beginning of 2025, investors have poured nearly $15 billion into the biotech industry. Some the the most focused areas of drug research are in Alzheimer’s, obesity, and cancer.
With new technologies being invented, primarily in the area of artificial intelligence, also known as machine learning, the time and cost of bringing a drug to market can be greatly decreased. Generally speaking, there are three main areas of machine learning are supervised, unsupervised, and reinforcement learning:
- Supervised Learning: This type of machine learning uses labeled datasets to train models, where both input and output are known. The model learns to map inputs to outputs and predict outcomes based on the training data.
- Unsupervised Learning: Unlike supervised learning, unsupervised learning deals with unlabeled data, allowing models to discover patterns and insights without explicit guidance.
- Reinforcement Learning: This type of machine learning involves an “agent” interacting with an environment and learning through trial and error, receiving rewards or penalties for its actions.
We take a hybrid approach to machine learning where both supervised learning and reinforcement learning are used in tandem to perform calculations and generate super-intelligent molecules. Reinforcement learning differs from supervised and unsupervised learning paradigms as it does not require a training set, instead, it learns by interacting with an environment, receiving feedback through rewards, and optimizing actions to maximize cumulative rewards. We incorporate reinforcement learning into our process for optimization of specific molecular properties. We perform quantum chemistry calculations and are able to manipulate the properties which directly affect the ADME (adsorption, distribution, metabolism, and excretion) of a compound. For example, the initial stages of drug discovery rely on virtual screening of molecules, or ligands, that can bind to a target protein (i.e. the protein that is associated with a particular disease). The current public, and even private, molecular databases are limited. Having the ability to generate new compounds with focused properties can be very advantageous and enable a biotech company the ability to find unique molecules that can be tailor-made for specific diseases. These experiments are performed in-silico where a select group of molecules are synthesized and experiments performed with in-vitro and in-vivo assays.
The success of these assay rely on the ligands that are chosen in the in-silico steps. It is the in-silico part of the pipeline that can heavily benefit from several new technologies in AI, primarily with molecular generation and the ability to create new molecules that have focused properties specifically designed to lower toxicity and optimize how the compound is absorbed, distributed, metabolized, and excreted by the body. These ADME conditions are assessed through Cipherica’s pharmacokinetic and pharmacodynamic routines that determine the success of a potential drug. By adjusting and balancing the molecular properties, it is possible to create super-smart ligands that will have the best chance for success in the clinical environment.
Biotech companies can burn through millions of dollars in a flash. It’s not uncommon for clinical trials to cost hundreds of millions, and to bring a drug across the finish line can easily approach into the billions of dollars. Even with these steep price tags and significant risk of failure, many investors keep the cash flow coming. However, it will be the biotech companies with the most intelligent drug discovery pipeline that will have the best chance of bringing a new drug to market. Cipherica concentrates on the upfront in-silico pipeline that will discover the lead compounds possessing the potential to be the most successful throughout the entire life cycle of clinical trials and FDA approval.
The Food and Drug Administration (FDA), a US federal agency commissioned to ensure public health, is the gatekeeper for all novel drugs. No biotech companies, or anyone else, can release new medicines without the approval of the FDA. It takes an average of 10-15 years to complete the FDA drug-approval process. It is at the in-silico stage that, taking from 2-5 years that can be drastically reduced in time, but more importantly, this is the stage where the most intelligent molecules can be chosen that will have the highest success rate.
Below, the graph outlines FDA trials process in years to show the meticulous nature of each phase:
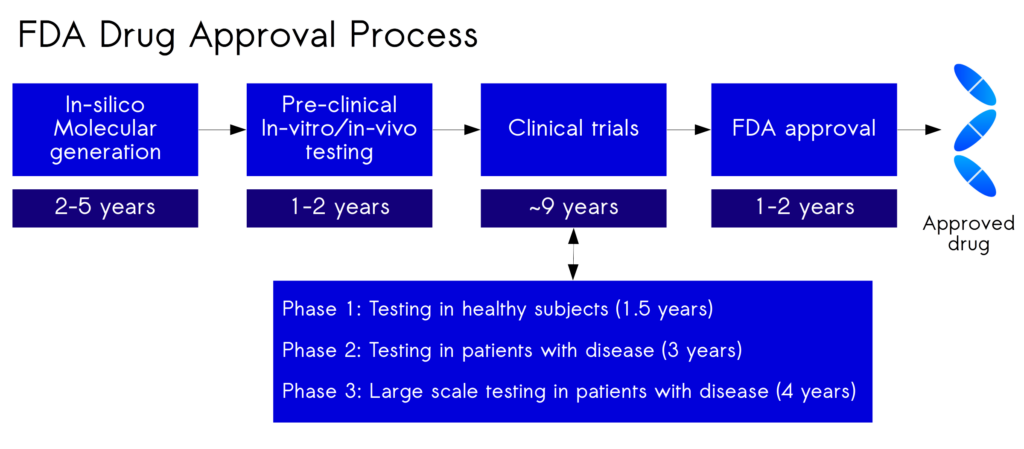
Although some innovative startups have managed to develop drugs at mush faster rates, in less than 18 months, it’s incredibly rare and not at all the norm. To put this into perspective, only about 30% of the Phase 2 candidates ever reach a Phase 3 trial. A failed clinical trial is financially painful, costing somewhere between $800 million and $1.4 billion.
Since the early 2010s, only about 10% of Phase 1 compounds have ever seen Phase 2. It’s worth pointing out that no other industry operates under such a significant failure rate. On a more positive note, compounds that make it to Phase 3 have a historical success rate of just under 50%. Fortunately, the success rate is increasing for this phase.
Nevertheless, Phase 2 is undeniably the point that testing weeds new drugs because of efficacy or safety. It is at this phase that Cipherica’s technology can make the most impact and generate compounds that have been designed specifically with pharmacokinetics. Thus, enabling the creation of compounds that can have the most success at making it across all phases and into the clinic. This stage in the process is a tell-all. Even with a bleak outlook, Phase 2 holds massive opportunities for biotech companies when it comes to funding and success. Cipherica’s drug discovery pipline, dbCIPHERTM, make’s it possible to create the greatest potential for success during each phase of the clinical trial to bring a potential drug across the finish line and into the clinic.